AI That Enabled Modern Conveniences Is Now Revolutionizing Cancer Drug Development
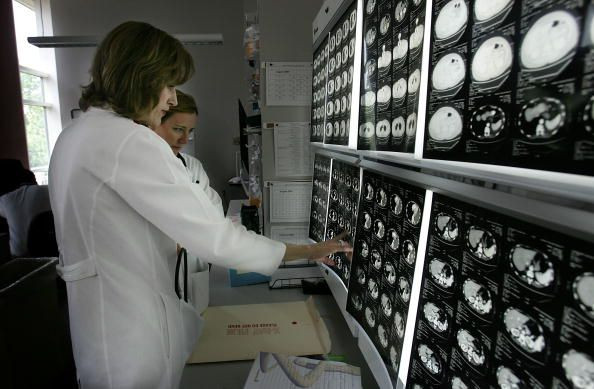
Two of the trendiest phrases in the technology space right now are artificial intelligence (AI) and machine learning (ML). Billed as being capable of forever changing how humans organize and understand data, the possibilities seem endless for social platforms like Facebook and Twitter, as well as products like Tesla or Alexa.
But these are not the only ways predictive technology can change lives. In some industries, such as biotechnology and life sciences, artificial intelligence and machine learning are actually being used to "save" lives, laying the groundwork for treatments for patients who aren’t even born yet.
For years, the pharmaceutical industry has conducted clinical trials for drug candidates by testing them on humans, some with terminal diseases, bravely serving as patient pioneers to determine a drug’s efficacy. Many of these trials are structured quite basically: if you’re developing a prostate cancer drug, you are likely testing it on about a hundred prostate cancer patients. The work is long and tedious, and often lacks precision, as some patients are more likely to respond to a drug than others.
But what if we could pre-determine our patient pools to better test who should experience an improved outcome from a cancer treatment?
Mining genomic and drug efficacy data from previous and ongoing cancer trials is one approach where biotechs and pharmaceutical companies can harness the power of AI to realize the goal of truly targeted oncology treatment. With recent advances in AI-enabled software, once unreadable genomic data sets that were simply too large to analyze with traditional statistical tools can be exported from clinical trials and entered into public databases for data scientists to access.
By mining patient data from prior trials, data analysts have the ability to stratify patient groups into subgroups and determine if any groups responded to the oncology therapies better than others, and further uncover gene signatures correlated to drug efficacy.
Better understanding the importance of a patient’s genomic signature in drug screening goes far beyond highly technical and scientific data.
Identifying gene signatures has clearly demonstrated the ability (and vast potential) for predicting the effectiveness of an oncology drug, and ultimately increase the likelihood of a drug successfully treating a stubborn or rare cancer. Many forms of cancer are complex, and within each type of cancer, there are shared genetic abnormalities that could be targeted with drug treatment.
The National Institutes of Health (NIH) report that scientists know the genetic basis for over 4,500 diseases, but only 250 of those have effective treatments using this genomic information (WSJ 2012). Never before has there been so much knowledge about genomics and complex computing techniques such as AI, where millions of data points can be analyzed with conclusions drawn within minutes.
Within this context, biotech companies can now enter at a later stage of development with abandoned or shelved drugs and design new pre-clinical studies to be executed with the stratified population as the new target. This increases the likelihood that a clinical trial will be successful in targeting the right patients with the right drug.
Why is this so important? Well, the pharmaceutical industry needs help. It is universally recognized that drug development is costly, risky and time-consuming. The median cost of developing one cancer drug is $648 million and the process often takes over seven years!
These costs translate directly to patients and consumers; the cost of one year of cancer treatment for one patient with one of these drugs is between $100,000 and $200,000 on average. With variable efficacy rates in cancer treatment, high drug prices, long development timelines and a lack of targeted therapy, patients and caregivers are negatively impacted by an old-fashioned approach to oncology drug development. This gap in efficacy and efficiency is a perfect problem area for an AI-enabled approach. There are thousands of abandoned and failed molecules to which biotechs could apply modern AI approaches to find patient groups that would benefit, and to improve the cost curve of the drug development process.
And yet, the very industry that discovers and develops oncology drugs to treat cancer has not embraced advances in AI and machine learning as other markets have, until recently. Making AI-powered changes to the drug development industry can switch the cost curve to one that will favor not only patients, but the further advancement of cutting-edge changes in drug development.
Technological advancements and quality of life are on a collision course, creating a great opportunity for industries in which there are great room for improvement, at the forefront of which is drug development. The capacity to process data today is greater than at any other time in history.
By allowing AI, genomics and drug development to collide, the benefits will be realized by patients, physicians, caregivers and investors. This is the time to capitalize on the opportunity and have a seismic impact on the standards we should all have for drug development efficacy, cost and timelines.
(Panna Sharma is the CEO of Lantern Pharma, a clinical stage oncology biotech company leveraging AI and genomics for oncology drug development.)
© Copyright IBTimes 2024. All rights reserved.