Dataminr Solves Twitter's 'Needle In Haystack' Problem For Hedge Funds And Banks
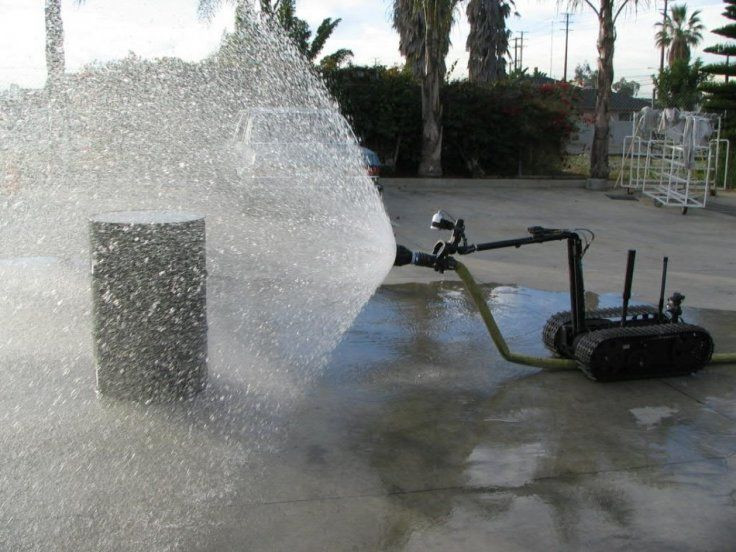
Dataminr is one of the few companies able to handle the entire publicly available Twitter dataset (known as the firehose), using the platform's wide swath of data to build smarter algorithms and better real-time alerts for its clients. Today, Dataminr says the learning curve from complete real-time data access over years is key to how it services business verticals in finance, corporate security, news gathering, and within the public space.
The company has found a way to negotiate the "needle in the haystack"' problem, which faces anyone who tries to process and store such a large volume of data.

Spanning the entire range of public content, Dataminr provides its clients with alerts from accounts they would otherwise certainly be unaware of. Steven Schwartz, Dataminr's president of news, uses the recurring food scare at Mexican restaurant chain Chipotle as an example.
He said: "Our users in the finance space interested in tracking Chipotle as a company, or more broadly that sector, were notified very early when a random Chipotle consumer tweeted about his friend getting extremely ill after eating in a Chipotle restaurant.
"Using our algorithm and data science, we were able to surface that alert and provide those clients the first notification that Chipotle was continuing to have food-related illness problems.
"After an alert is sent, Dataminr continues to track emerging events like this, and we detected additional relevant information in the following minutes and hours that indeed revealed this to be a broader issue for Chipotle. Chipotle's stock subsequently dropped.
"How would you ever know to follow that particular individual, who probably only had a few hundred followers on Twitter, who may or may not have even used a hashtag or a stock ticker in the tweet? Our service solves that challenge."
Over time Dataminr has learned certain patterns around how information emerges when people first talk about things on Twitter, and how particular information surfaces. Dataminr is different from a lot of other companies in the social media space, which are analysing sentiment that can be used to make tradable decisions, for instance.
Schwartz said he often hears from people who have endeavoured to take a slice of the dataset and try to apply some sort of data science to it. "They end up getting fractional value and results. We love talking to them. They know the value and power of the data set, yet they have not been able to harness it themselves."
Back in 2009, the social media landscape looked a lot different than it does today, notes Peter Bailey, chief strategy officer, Dataminr. "Twitter was scaling globally as a unique public platform where individuals around the world could Tweet about breaking events they were witnessing. Essentially our bet was that this behaviour would continue to flourish on Twitter and it did. We built a real-time system to detect this information by identifying unique data patterns that emerge on Twitter when breaking information is first published by Twitter users anywhere around the world.
"You never know who might eat tainted food at Chipotle, yet financial professionals who cover that sector need to know that information when it happens. Dataminr's system was built to understand how financially relevant events like food-borne illness outbreaks have emerged on Twitter in the past, and our system can detect such patterns in real-time, determine relevancy, and alert our clients. Over time, our machine has improved its ability to unearth and discover financially relevant information.
"We don't provide a sentiment score or market ourselves as a sentiment company. There are other companies who are trying to provide a social media sentiment score or indicator; those efforts are understandable and some people do it better than others.
"That said, we deliver context and information around financial topics that can help inform sentiment. There is an aspect to our service that allows for more proactive research. Users can analyse trends and apply their own expertise on whether they are repeatable, and whether that might be a bullish or bearish indicator for a stock."
A problem when trying to detect signals that can be traded on out of a sea of noisy social media data is the high probability of false positives.
Bailey said: "There are situations on Twitter that are rumours, false statements, and mis-statements. We have been able to study those patterns too and can recognise events which are forced or manipulated versus events that are more authentic. Still, our clients want to know about it if it may impact their portfolios so we are pushing differentiated, relevant content in real time.
Bailey said early on in beta testing, Dataminr's finance customers were asked how early they would want to receive information, taking into consideration that something may happen on Twitter that Dataminr generates an alert about, that may not ultimately turn out to be a globally impactful event.
"Our users told us they wanted to know about information as early as possible because markets can move off of all kinds of information. We have done a lot study of false information on Twitter and information that is clearly forced or manipulated."
Schwartz added: "I have never heard of a client that is angry with us because they made a decision and it went awry for them. I think everybody across all industries understands that whether it's social media or a more traditional source of information, people are looking for context; whether they make an immediate decision or wait for that second kind of validating point is up to them and their work flow."
This article was first published on Aug. 18, 2016.