Google Creates Artificial Intelligence Program Smart Enough To Learn From Its Mistakes
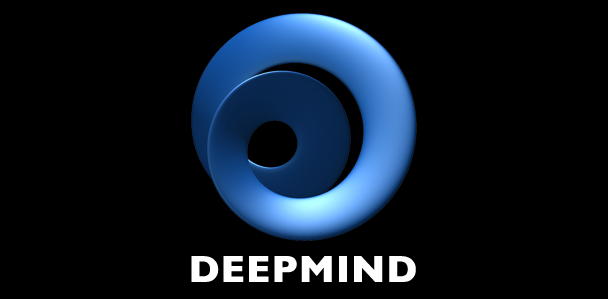
An Artificial Intelligence (AI) program designed by Google has taught itself to play and win video games, performing better than humans in several Atari games from the 1980s. The development is being hailed as a significant advancement in the field of “deep learning,” which aims to create machines capable of mastering a diverse array of challenging tasks.
Although computers have, in the past, mastered complex games to a level where they have bested human competitors -- IBM’s Deep Blue famously beat world champion Garry Kasparov in 1997 -- what makes this development stand out is that it is the first time that a system has actually learned from experience and adapted in real-time to unexpected developments. The program, named Deep Q-Network (DQN) by the developers at Google’s DeepMind division, is the first computer program to teach itself to succeed at tasks after starting from scratch and learning from trial and error -- much like humans do.
DQN, which was provided almost next to nothing in the way of instructions, was presented with 49 different Atari video games, such as Breakout, Pinball, Space Invaders and Pong. After several rounds, the researchers found that the program performed better than humans in 29 of the games, and in some games, like Pinball, it did 26 times better -- using moves that no human had ever tried.
“The only information we gave the system was the raw pixels on the screen and the idea that it had to get a high score. And everything else it had to figure out by itself,” Demis Hassabis, DeepMind’s vice president of engineering, reportedly said.
To a computer with no pre-existing knowledge of what the arrangement of raw pixels signifies, the data it receives from these games is meaningless. However, what DQN did was to intuitively analyze the shape, size, color and arrangement of these pixels so that it eventually understood what it was looking at.
“On the face it, it looks trivial in the sense that these are games from the 80s and you can write solutions to these games quite easily,” Hassabis reportedly said. “What is not trivial is to have one single system that can learn from the pixels, as perceptual inputs, what to do.”
Moreover, the program also has the ability to analyze its previous actions that led to better scores and learn from its past mistakes. However, when it came to games like Pac Man and Montezuma's Revenge, which require a certain level of pre-planning, DQN did not perform as well as human players.
“One of the things holding back robotics today, in factories, in things like elderly care robots and in household-cleaning robots, is that when these machines are in the real world, they have to deal with the unexpected. You can't pre-program it with every eventuality that might happen,” Hassabis reportedly said, adding that the next step in their research would be to test DQN with more complex data.
“Ultimately, if the agent can drive a car in a racing game then, with a few tweaks, it can drive a real car,” Hassabis added.
The program might one day be used in Google’s self-driving cars that would have the ability to learn how to drive without needing preloaded maps. However, the creation of such systems, which have a keen awareness of their surroundings, is still a long way off.
© Copyright IBTimes 2024. All rights reserved.