How Can Finance Catch Up With Other Intelligent Real-Time Systems?
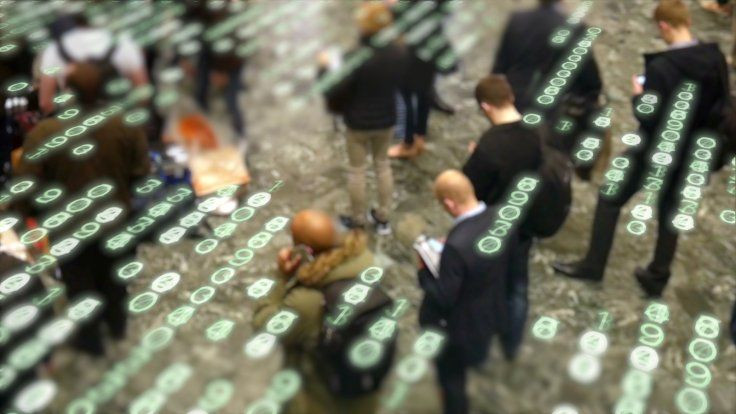
When it comes to bringing intelligence to real-time engineering systems, the world of finance has been hindered by its legacy. Compared to things like self-driving cars, incumbent financial infrastructure takes a very long time to update, and is siloed into systems that cannot really talk to each other.
Paul Bilokon, founder of Thalesians, an organisation to promote deeper thinking and philosophy within finance, points out that many non-financial systems are using software techniques that are far ahead. But he also sees this changing thanks to improved infrastructure tools and advancements in machine learning within finance. Paul will be speaking about new infrastructure and showing off some machine learning libraries at the forthcoming IBT data science and capital markets event.

He said: "Look at all these techniques that people use outside finance. For example, Facebook, Google all these organisations, even the telecoms; there is a need to respond to change rapidly and update and maintain correct state internally. They are all using techniques and software methods which are way ahead of finance."
Bilokon believes that in the last couple of decades before the credit crunch, finance went in the wrong direction of increasing complexity in things like derivatives modelling, to the extent that people didn't really understand what was behind this complexity – which in some senses served the old banking world. "I think we are coming back to some sanity and understanding. We need to build systems that serve people," he said.
Bilokon, who built electronic trading platforms for Deutsche Bank, says what happened in derivatives there was huge over-complication in microscopic modelling. "Nobody really understood at the macro level how the economy based on derivatives really functioned because people just didn't have enough data. I was really shocked that most derivatives quants had never heard about backtesting. Much theoretical thinking is not supported by empirical work.
"There is simpler modelling and there is emphasis on robustness, and there is also emphasis on the operational aspects: how margining works, how credit valuation adjustment works.
"But if you were to look at a typical bank, from the practitioner's point of view, you would have disparate quant teams working on multiple libraries which are hugely complex. Then if you want one system to start talking to another that becomes very problematic."
Bayesian Learning
Advances in optimisation are being driven by techniques like Bayesian Learning, complemented by technological advances in terms of infrastructure; projects like Apache Spark; kdb+ and q language.
Bayesian Learning has been described as a model for logic in the presence of uncertainty; a method which matches human intuition. To evaluate the probability of a hypothesis, the Bayesian probabilist specifies some prior probability, which is then updated to a posterior probability in the light of new, relevant data.
"This is software that maintains as its state degrees of belief as numbers. So that is what machine learning really is.
"These techniques are very powerful. Take defence, intelligence, even e-commerce. Amazon stocks and prepares your order days before you have actually ordered it. That's because they use Bayesian learning methods to update information on behavioural trends. Similarly, cameras can track objects using particle filtering methods and predict their future locations."
As far as financial technology is concerned, portfolio managers today use a combination of techniques. "People are using everything," said Bilokon. "As a portfolio manager I often used things like ordinary least squares, because the very first step in much data analysis would be to do a regression."
He added that a healthy degree of discretion is always needed because the system will only do fundamentally what you tell it to do. It can learn from data but it cannot get information out of nothing.
Certain insights come from a leftfield position beyond the context being modelled. To take an example from physics, an understanding of classical Newtonian mechanics is changed by the realisation that at certain times scales, or a certain size scales, quantum effects come into play.
"You couldn't learn this from the context," noted Bilokon. "This insight comes from somewhere else. So in some situations you need to update your thinking. This is very much more the case in human systems where there are massive interactions between different economies and so on.
"Whatever your context is, there will always be something that is bigger that is coming out of that context, that you haven't really thought about, that your system doesn't know about.
"So discretion, experience, intuition are very important. I do design for automation, but I'm not sort of naive enough to say, this is going to be doing everything on its own forever. Things will always be changing," he said.
This article was originally published on Dec. 1, 2016.