Open-Sourcing Code, Collaboration — Fintech — Changing Financial Services Industry
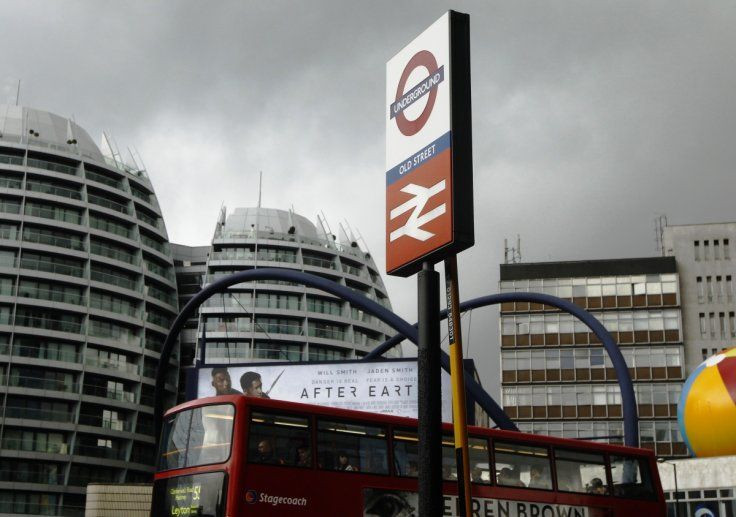
Within the silos of incumbent financial services, so-called fintech companies are good at picking off one thing only and doing it well.
This approach is also taken within data science where a lot of the properly intelligent work is about understanding the domain (problem) and how best to use the information/data for the problem you have. In doing so, a fintech approach — collaboration, open-sourcing code — is helping to gradually change the culture of finance, even in some hitherto heavily guarded domains.
"Without this specialization and domain knowledge, it's very hard to rise above the noise. However, the algorithms themselves are often applicable in many areas or problems, and we are probably seeing decreasing specialization here," said Dr. Tristan Fletcher, research director of Thought Machine.
"Fintech lends itself particularly to specialization because there are many well-packaged problems that need to be solved and can be clearly delineated — KYC [know your customer], AML [anti-money laundering], credit checking, etc. Having a competence in one area does not necessarily imply it in others, so it is harder to justify being a corporate fox versus a hedgehog."

Bonus-driven executives with a big bank or fund might be reluctant to share their secret sauce with people in their own company, nevermind other corporates. In contrast, within the majority of small fintech startups, most employees will share (to varying degrees) in the ownership of the company, which will drive collaboration and the sharing of ideas internally. Furthermore, because of the relative balkanization of a lot of the domains in the fintech ecosystem, there is much more incentive to co-operate than be competitive in the industry.
"I think the main reasons for the disparity between fintech and investment banks and hedge funds is that a lot of the sources of revenue in the latter are closer to a zero-sum game. Profiting from pure speculation, for example, means that someone else must be losing money [assuming the counterparty isn't doing something more economically useful like actually mitigating risk]," Fletcher said.
"Furthermore, compensation in traditional financial institutions is much more closely linked to one's actual proximity to [often larg] amounts of money. It's often a food chain with front-office staff getting more than middle office, getting more than back office for example.
"Incentivization through cash bonuses, which is gradually going out of fashion, encourages a very short-termist approach. Fintech incentivization, being a relatively new and entrepreneurial sector, is much more about ownership in the company, which is clearly much longer term.
"Value in the fintech world is much more about the execution of an idea, whereas hedge funds, for example, are known to take a cloak and dagger approach to their intellectual property, in order to justify their management fees."
However, some financial institutions and even secretive hedge funds are changing, sharing information and open sourcing some noncore infrastructure. A good example is the U.S. hedge fund AQR, which open-sourced some of its infrastructure around time series storage and helped kickstart the pandas libraries community.
"I have heard of more and more private companies open sourcing noncore infrastructure and this makes a lot of sense: It adds credibility from a technology perspective to the organization, gets lots of developers to critique and improve any code at very low cost and encourages the adoption of any software the company might be pushing out," Fletcher said.
As much as fintech is providing an exciting new lease on life for the financial services industry, there is a super-abundance of hype out there. Terms like data science, artificial intelligence [AI], machine learning, blockchain and so on have become extremely hyped terms. Attaching these words to your LinkedIn profile if you work in software will get your profile more views; framing a project in this way can get funding more easily.
"There are a lot of people claiming to be doing artificial intelligence and machine learning purely to get funding or generate publicity. In some cases this is being a little bit disingenuous, using machine learning when something simpler would be fine, and in others, it's just bare-faced lying. This obviously doesn't just apply to start-ups," he added.
"The danger is that you get disappointments that blemish the entire field: Perhaps some unscrupulous people use machine learning in a massively incorrect way and you get a well-publicized failure that tarnishes the reputation of the entire genre."
Fletcher said there are no easily recognizable conditions that prove some entity is genuinely doing this sort of work, but he would generally look to see whether some of the senior staff did at least have some strong academic credentials in AI or statistics.
The financial arena is characteristically conservative, and when it comes to using AI, nonfinacial use cases associated with the likes of Google DeepMind and IBM Watson (which are modeled around potentially replicating human behaviors) garner much more attention and would appear to be far ahead.
Fletcher added: "One of the problems with AI/deep learning is interpretability; it's hard to work out what the black box is actually doing. And this is dangerous in applications which could impact the tightly coupled financial markets or indeed people's bank accounts.
"A lot of the machine learning in markets is based around time-series analysis, and it is more typical to use different methods [less related to biological processes] here.
"There has been work using genetic algorithms, biologically inspired in a different way, on portfolio construction [for example bond portfolios by a product called Galapagos] and I'm sure many people used Neural Nets in the '80s but a lot of the ML [macine learning] methods used in markets nowadays are very far away from being biologically plausible in any way."
© Copyright IBTimes 2024. All rights reserved.