Planet Or Not? Researchers Develop New, Faster Method Of Confirming New Planets
KEY POINTS
- Scientists developed an algorithm that can determine real planets from false positives
- The new method is much faster than previous techniques
- Using the method, the researchers were able to confirm 50 new planets
- The method can be useful in scouring data from planet-hunting missions
Scientists confirmed 50 new planets using a technique they developed. The method is said to be much faster than previous techniques and, could help determine which planet candidates are actual planets and which are not.
In the search for exoplanets, many surveys use what's known as the transit method wherein possible planets are detected by the dips in the brightness of a star, signifying that an object has passed in front of it. The problem is that these "dips" could also be caused by a binary star system, another object that's not really a planet or, even possible errors in the camera.
To remedy this problem, a team of researchers developed an algorithm that could help sift the massive amounts of planet candidate data to find the real planets. The method is based on machine learning, which is a form of artificial intelligence, and they trained it to recognize real planets using large data samples of confirmed planets and false positives from the Kepler mission. Once trained, it can be automated so it can sift thousands of candidates in a short amount of time.
"Our models can validate thousands of unseen candidates in seconds once applicable vetting metrics are calculated, and can be adapted to work with the active TESS mission, where the large number of observed targets necessitates the use of automated algorithms," the researchers wrote in their study published in the Monthly Notices of the Royal Astronomical Society.
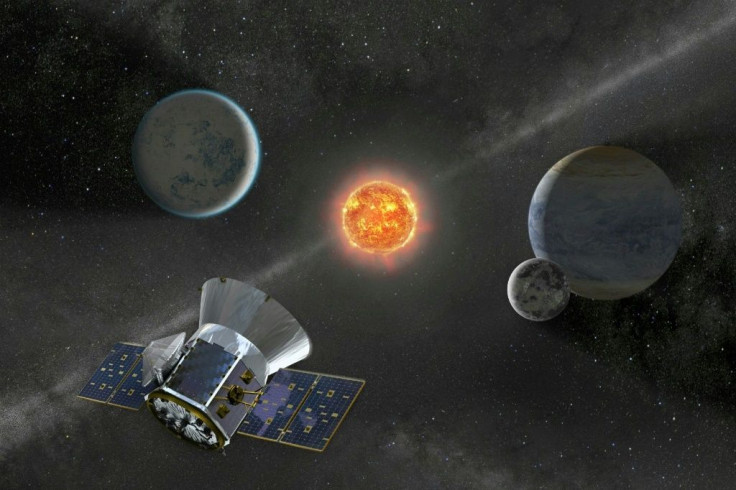
Compared to previous methods, the researchers' method not only ranks the planet candidates but determines their probability of being real planets. Such a method could help missions and studies to determine exactly which planets to focus on once they're confirmed instead of looking at objects that still may or may not be planets.
The researchers themselves were able to use their automated algorithm to confirm 50 new planets out of the unconfirmed candidates from the Kepler mission, so these planets can potentially be added to the list of planets that telescopes can focus on.
"Almost 30% of the known planets to date have been validated using just one method, and that's not ideal," study lead Dr. David Armstrong of the University of Warwick said in a news release from the university. "Developing new methods for validation is desirable for that reason alone. But machine learning also lets us do it very quickly and prioritize candidates much faster."
With potentially thousands of planetary candidates so far, an algorithm that can effectively and quickly sift the data for real planets would be incredibly useful in analyzing the data from planet-hunting missions such as NASA's TESS.
"Fast, automated systems like this that can take us all the way to validated planets in fewer steps let us do that efficiently," Dr. Armstrong said.
© Copyright IBTimes 2024. All rights reserved.