Prescriptive Analysis In Manufacturing To Boost Profitability
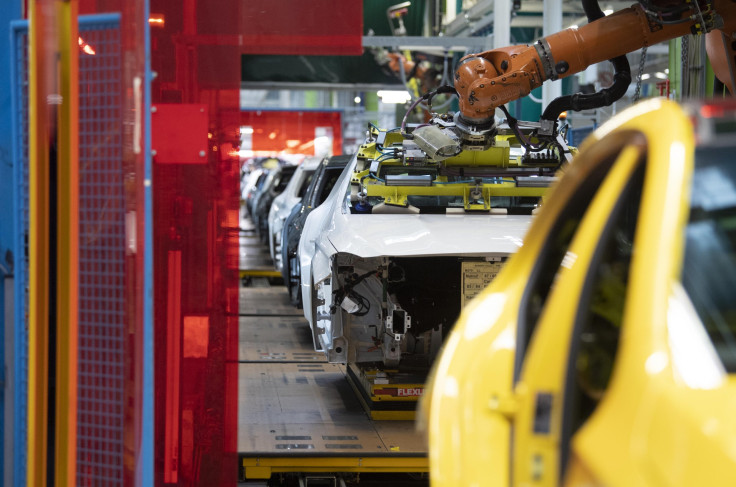
I have to chuckle when I hear people ask, “Did you know analytics can be applied to manufacturing?” Well, yeah — have you ever heard of operations research? It’s been around since World War II and is the use of data, mathematical models and algorithms to solve problems in operations. Sound familiar? I suppose some people think of analytics as new to manufacturing because of analytics’ focus on big data, social media and marketing. Although many might disagree, I equate analytics with operations research/management science, especially in its origins, which were problem-centric. Analytics has a lot to offer manufacturing, and in some ways, manufacturing is ahead of other sectors in the use of analytics - there is plenty of opportunity for manufacturing to leverage analytics beyond traditional planning and scheduling.
The analytics toolbox
There are several classifications of analytical tools, but the most common categorization comprises of three buckets: descriptive, predictive and prescriptive analytics. Descriptive analytics includes descriptive statistics, data queries, data visualization and data dashboards. These tools are generally used to describe what has happened. Predictive analytics includes time-series forecasting models, various types of regression analysis, and predictive data mining and machine learning. Predictive analytics uses past data to predict the future or to ascertain relationships between variables of interest. Finally, prescriptive analytics differs from descriptive and predictive analytics -- prescriptive analytics prescribes a course of action for the manager. For example, optimization, heuristics and other rule-based systems are prescriptive.
In the context of manufacturing, a control chart is descriptive; a logistic regression to predict machine failure is predictive; a scheduling algorithm to minimize changeover cost is prescriptive. Of course, no one does descriptive and predictive analytics just for fun. By putting rules on top of descriptive and predictive analytics, they become prescriptive -- that is, they help you solve a problem. For example, a descriptive control chart becomes a prescriptive tool when it is combined with a rule that says, “if there are five consecutive data points outside of the control limits, stop the machine for inspection/recalibration.” Analytics is problem-focused, so all of it contributes to problem-solving. In a sense, the goal of analytics is (or should be) prescriptive.
Manufacturing and prescriptive analytics
How can manufacturers leverage prescriptive analytics? Maybe your industrial engineers have already solved your quality control, inventory and production control problems, but they are thinking of new sources of data that would allow for even smarter operations? And are they working with the marketing team to develop new and innovative products and services that leverage all available data? Finally, what role might artificial intelligence play in your operations?
Smarter operations: investing in data and connectivity
Is everything in your operations as connected as it could be? How would investing in sensors and/or smart tags in various stages of your operations provide data that could be leveraged to take advantage of prescriptive analytics? For example, can investing in sensors in your machinery enable models improve the accuracy of your predictive maintenance that can then enhance the effectiveness of optimization models to proactively schedule the maintenance? The result would be an increase in capacity. Can better data from smart tags lead to even better inventory control and order picking efficiency that, in turn, will allow workforce optimization? The result could be a reduction in overtime costs. And how connected are your systems to those of your suppliers? Do you constantly collect data on supplier performance? Can this data enable supplier optimization that would allow you to better control costs and mitigate risk? Should you create a combinatorial auction with your certified suppliers that would lead to significant cost reductions? All of these examples have one thing in common -- an investment in data that can then be leveraged through prescriptive analytics.
Product innovation: new goods and services from your product data
Marketing has probably been the dominating focus of the modern analytics movement. Therefore, it should not be surprising that the manufacturing-marketing interface is ripe for the innovative use of prescriptive analytics. Similar to making your manufacturing/machinery smarter, can you make your product itself smarter in a way that generates new opportunities? Agricultural equipment manufacturer John Deere and aircraft engine manufacturer GE Aviation both now place sensors in their products to help their customers better manage their equipment. The sensors in John Deere tractors send data to John Deere, which then offers prescriptive analytics as a service -- how to better maintain the equipment, how to save fuel and, when coupled with weather data, how to operate to increase yield. Similarly, based on data collected from its engines, GE Aviation offers prescriptive analytics as a service to help its customers with preventive maintenance and prescribing better glide paths for fuel efficiency. How can you collect data from your products that can be used to provide a new valuable good or service for your customers?
Automation through artificial intelligence
Artificial intelligence (AI) is currently a hot topic in all areas of business, especially in manufacturing. AI can be either predictive or prescriptive analytics. The key is that AI is typically automated and updates its task in real time with new data. These real-time updates can be for prediction or prescription. In the latter case, AI can be used for fast real-time decision-making most effectively for operational decisions rather than tactical or strategic ones. For example, when coupled with machine sensor data, AI could be used for predicting and then scheduling preventive maintenance of the machinery. As more data enter the system, and through learning, AI could also be used to prescribe an adjustment to the machine in real time based on quality control data.
Investing in analytics
All of these examples of prescriptive analytics in manufacturing require an investment in data, connectivity and ultimately, smart analytics professionals. To remain competitive in the low-margin retail grocery space, The Kroger Co. has invested heavily in technology and analytics with great success. Kroger used simulation and technology to cut the average wait time of its customers from about 4 minutes to 26 seconds. Kroger is using analytics to drive online shopping, a better customer experience, and better product mix and availability. The title of a recent Wall Street Journal article says it all: “Every Company Is Now a Tech Company.” How will you invest in technology and analytics to remain competitive in manufacturing?
Jeffrey D. Camm is Associate Dean of Business Analytics and Inmar Presidential Chair in Analytics at the Wake Forest University School of Business. He is a Fellow of the Institute for Operations Research and the Management Sciences and co-author of the third edition of Business Analytics.
© Copyright IBTimes 2024. All rights reserved.