Why Specialized Models Are The Future Of AI For Business
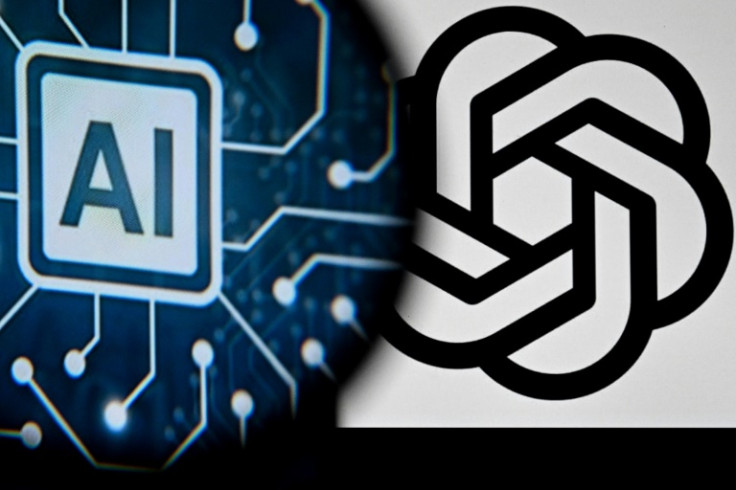
In many areas of the business world, AI use is already taken for granted. Today, as the AI ecosystem is maturing, many companies that have specialized use case needs are moving on from the first generation of generic GenAI LLMs to become more choosy about the models that they use.
This is driving a rise in organizations developing their own models, while vendors are responding to demand with a greater range of models each of which is more niche-focused and specialized. According to one recent study by TCS, 51% of CEOs said that their company plans to build its own LLM to meet its own business needs.
Apparently, specialized AI models for niche use cases are the new adoption trend in business AI.
Specialized knowledge and use cases
Many businesses are discovering an awkward truth about generic LLMs: they may be good for basic, casual use cases, but their performance can be poor when it comes to specialized use cases. For example, tasks like summarizing and preparing legal documents require AI models that have been trained on legal jargon. A generic LLM simply won't understand the legal terms in any depth that professionals can rely on.
"So maybe there's an LLM that is tuned for accounting, another one is tuned for marketing, maybe an LLM is tuned on healthcare, maybe an LLM is tuned on insurance data," explained Avi Perez, CTO at Pyramid Analytics. "Therefore, it makes a lot of sense that you're able to switch out the LLM based on the data that you're looking at." Pyramid's AI business analytics solution is built for use with multiple LLMs, so that users can connect it to whichever model makes the most sense for the analysis they're working on.
The wider ecosystem has already seen success stories with Perplexity Pro, considered by many to be the best for "vibe coding" use cases. Frase is hailed for the quality of the articles it generates. BloombergGPT, an AI model trained on financial data, supports market analysis for investors.
There's also a need for specific models for different languages, and various types of architecture are more effective for different use cases. For example, LLMs like ChatGPT are excellent at summarizing content, but less effective at data handling. Newer models are being developed that can handle large datasets and manage data more efficiently.
Real-time updates and rapid response
When it comes to AI models, there's a constant tension between speed and throughput. Low latency models deliver faster answers, but they can't handle massive datasets. On the other hand, high throughput models can crunch enormous datasets, but you have to wait longer for the output.
Tesla's Autopilot AI model is designed for (somewhat) autonomous driving. It processes data from cameras, radar, and ultrasonic sensors to navigate roads, avoid obstacles, and assist with driving tasks, all at high speed.
Obviously, the ideal speed-to-throughput balance depends on the use case at hand. In situations where real-time information matters, you need a model that's optimized for retrieval augmented generation (RAG) functionality to pull data from external sources quickly.
HomeZada's Homeowner AI functionality uses RAG alongside external, proprietary, and user data to improve the accuracy and reliability of its licensed LLM and deliver personalized insights for homeowners for remodeling projects.
Indeed, AI agents and custom models alike require access to fresh, diverse data to ensure that the AI is capable of generating output that's trustworthy. Bright Data's enormous network of residential proxy IPs and agentic browser infrastructure can source data in real time from the internet, for precisely these contexts, while also integrating with data processing workflows.
Greater affordability and innovation openness
Training and running an AI model requires a lot of compute power. The bigger the model, the more processing and electricity you need, making price a powerful argument for customized, leaner AI models. That's particularly true for enterprises that run models on self-hosted architecture, which is necessary for data privacy.
Teams that want an effective AI model can afford smaller specialty models, which are effective in specific use cases that have precise datasets. As of this month, Microsoft is rolling out its Phi-3 small language models (SLM, as opposed to LLM), and Apple is releasing eight small language models for handheld devices.
The cost of using small models like these is close to zero, in contrast to LLMs with high monthly licensing costs or the millions of dollars needed to build your own.
Enabling access to AI models is also important to drive innovation, as Zeev Farbman, CEO of Lightricks, emphasizes. "If you actually want to build alternatives, APIs are definitely not enough," he recently told Venture Beat. "You need to give people—academia, industry, enthusiasts—models to tinker with and create amazing new ideas."
In order for non-profit researchers, imaginative startups, and passionate innovators to be able to tap into the power of AI models, those models have to be democratized, which is why Lightricks opted to release its LTXV model for video creation as a open-source code base optimized to work well on consumer hardware.
Superior data privacy considerations
Another set of circumstances that require smaller specialized AI models is when compliance is paramount and demanding. Enterprises that want to use AI models on their own sensitive proprietary data or customer data need small, self-owned, in-house models to meet compliance requirements.
Sharing sensitive information with publicly available, cloud-hosted models has been known to cause disastrous results, as this data can be used to train the model, and your information could leak. Even the act of uploading files via your browser can open you up to security vulnerabilities.
As mentioned above, smaller AI models are affordable enough to make them accessible even for smaller companies. These models can be hosted entirely on premises, and they can be trained on smaller datasets consisting solely of first-party information. This gives companies total control over how their data is used.
This was the motivation for Dayforce, a human capital management software vendor. Dayforce uses AI and related technologies for several functionalities, to match employees at client companies with career coaches, without breaching any strict regulatory requirements around using personal identifying information.
Specialized AI models could be here to stay
Although it's hard to make predictions in the fast-paced world of tech, there's every reason to believe that specialist AI models will be a mainstay of AI. Businesses find them more trustworthy, affordable, effective, fast, and compliant, making them top of the list for a range of use cases.
© Copyright IBTimes 2024. All rights reserved.