Is Crowdsourced Data Reliable?
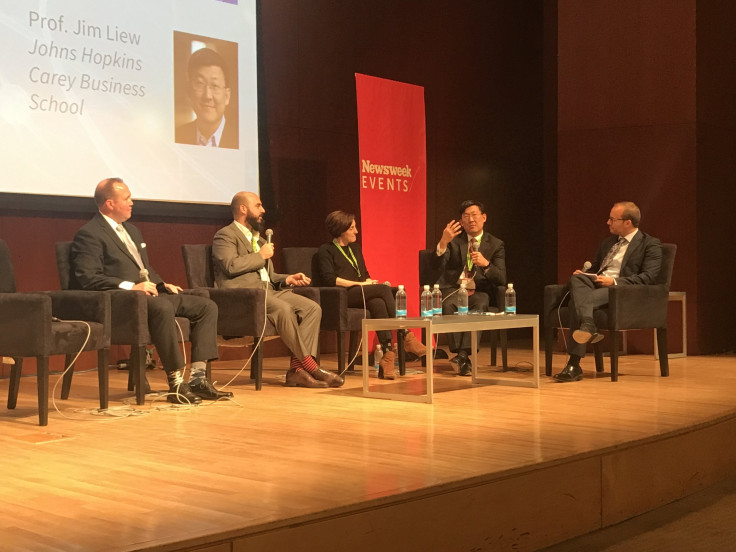
Countless companies now leverage crowdsourcing as a key component of their business model even though crowdsourced data has several crucial weaknesses. It’s hard to guarantee the diversity, reliability and expertise of information collected from strangers. For example, NBC reported more than 3,000 global news outlets inadvertently published tweets by Russian-backed trolls during the 2016 U.S. presidential elections. Crowdsourced data is often so dangerously flawed that this idea is now the premise of a CBS crime drama called "Wisdom of the Crowd."
When it comes to the financial industry, crowdsourced mistakes can be costly as well as embarrassing. So machine learning experts are busy figuring out solutions for dynamic prediction markets. Many of them rely on machine learning to manage human participants at some stage or another.
“If you allow anybody and everybody to come make estimates, how do you make sure somebody isn’t trying to game the system, that ridiculous people don’t put in ridiculous estimates?” Leigh Drogen, cofounder of the crowdsourcing fintech platform Estimize, said at the Artificial Intelligence & Data Science conference in New York City. “What we do is run a series of machine learning models against a set of rules...those models deal with weighing the contributors in different ways.”
Drogen found a few key variables were the strongest indicators of inaccurate predictions. It is often as simple as seeing how long the user spent on the page before entering an estimate and what patterns their profile history reveals. “A lot of people ask how we know what is going into people’s estimates. We don’t care because we can back into it via their behavior and statistics,” Drogen said. This strategy lines up with what the UC Berkeley students behind RoBhat Labs did to identify trolls and Twitter bots flooding social networks with propaganda. Within hours RoBhat Labs found around 6,000 bot accounts.
Artificial intelligence algorithms are great at identifying outliers. However, they are less proficient at highlighting unique “alpha” insight. Jim Liew, assistant professor of finance at John Hopkins Carey Business School, told International Business Times he thinks crowdsourcing is a good recruiting tool for hedge funds who want to tease out the “alpha” from untrained yet brilliant contributors. “Identify really smart users and create a research group with some kind of oversight...a lot of hedge funds already do this,” Liew told IBT. “You also need people to challenge each other...the best research that I’ve done is always with students.”
Liew said developing a catalyst relationship, in private, allows for financial better strategies. “I don’t think the crowd, at least the sorts of people who do this, are persistent enough to understand all the different sources of influence and drivers,” he said. “There’s not enough struggle. There’s not enough challenging of their models.”
Bloomberg reported the quantitative hedge fund WorldQuant, which manages more than $4.5 billion for Millennium Management LLC, operates the WorldQuant Challenge, which is an ongoing, global competition for building algorithms with 7,000 active users on its online platform, called WebSim. As long as this type of public engagement compensates contributors, diverse models could continue to spread throughout the industry.
Jessica Stauth, managing director of the investment team at the fintech startup Quantopian, added her own insights on crowdsourcing financial data to the New York panel. “You’ve got to have something that’s achievable, something where you can see incremental progress,” she said, describing a contest for analyzing dynamic markets. “Bring us your ideas and we will share the money with you,” agreed Morgan Slade, CEO of the crowdsourced algorithmic trading startup CloudQuant. “For us, engagement means breaking it down into a contractible problem.”
In short, crowdsourced data appears to work best when coupled with AI algorithms that weed out bad actors, plus thoughtful incentive strategies that apply to both open source collaboration and small group dynamics. Adaptability is also key, especially for predicting market trends. “Your algorithm has to be dynamic,” Liew said. “What worked for the last six months won’t work once everyone finds out about it.”
Editor's note: Newsweek Media Group and International Business Times partnered with Structure to host this week's Artificial Intelligence & Data Science event.
© Copyright IBTimes 2024. All rights reserved.