Science Breakthrough: Neural Network-Driven Approach Could Usher In A New Era Of Optical Astronomy, Researchers Say
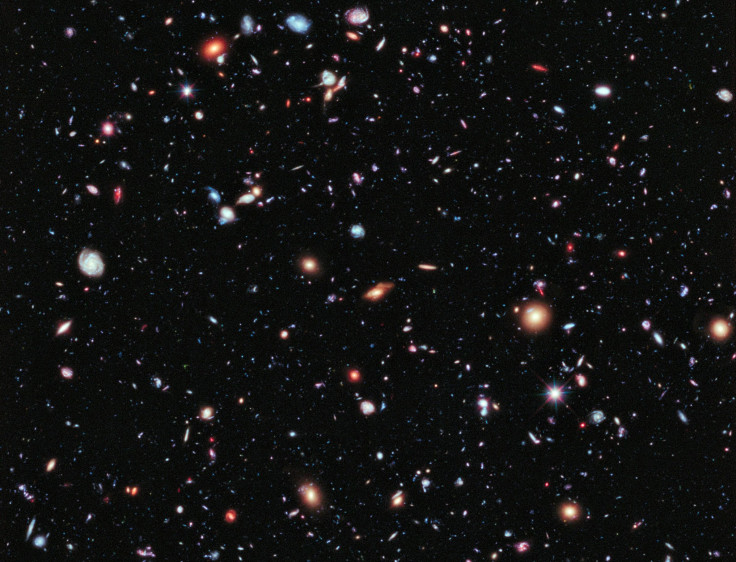
Neural networks — systems patterned after the arrangement and operation of neurons in the human brain — have driven some of the greatest breakthroughs in the field of artificial intelligence in recent years. AI powered by neural nets have not only excelled at tasks that require pattern recognition, they have also succeeded in performing tasks that require basic logic and reasoning — areas that have conventionally been computers’ Achilles' heel.
In this backdrop, the question is, can neural networks be exploited to usher in an era of data-driven astronomy and create some of the sharpest ever images of celestial objects? The answer, according to a new study published in the Monthly Notices of the Royal Astronomical Society, is yes.
In the paper, a group of researchers from the Swiss university ETH Zurich describe a neural net-enabled system that can recognize and reconstruct astronomical features that telescopes could not resolve, including star-forming regions, bars and streams of dust in galaxies.
In order to create this system, the researchers used an adversarial learning technique, wherein two neural networks compete and try to outsmart each other. By doing so, and by checking the images created by the neural nets against the original high-resolution image, the teaching program was able to drastically improve images captured in the early years of the Hubble Space Telescope.
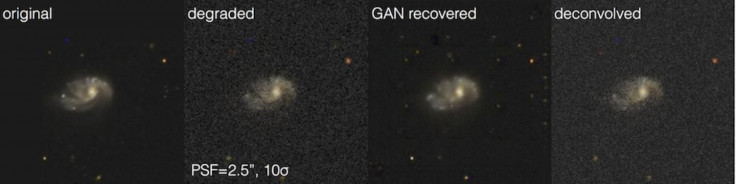
A similar approach has, in the past, been used by researchers at Massachusetts Institute of Technology to create a deep-learning algorithm that can, given a still image from a scene, create a video that simulates the most likely future of that scene.
“The massive amount of astronomical data is always fascinating to computer scientists,” study co-author Ce Zhang said in a statement released Wednesday. “But, when techniques such as machine learning emerge, astrophysics also provides a great test bed for tackling a fundamental computational question - how do we integrate and take advantage of the knowledge that humans have accumulated over thousands of years, using a machine learning system?”
Currently, the resolving power and sensitivity of telescopes is limited by the size of their primary mirror (Hubble has a 2.4 meter primary mirror, while the James Webb telescope, scheduled for launch in 2018, would have a 6.5 meter diameter mirror).
This is where the neural network described in the study is likely to play a crucial role.
“We can start by going back to sky surveys made with telescopes over many years, see more detail than ever before, and for example learn more about the structure of galaxies,” lead author Kevin Schawinski said. “There is no reason why we can't then apply this technique to the deepest images from Hubble, and the coming James Webb Space Telescope, to learn more about the earliest structures in the Universe.”
© Copyright IBTimes 2024. All rights reserved.