Wolfgang Lück on How AI Will Accelerate Saving CubeSat Data from the Digital Graveyard
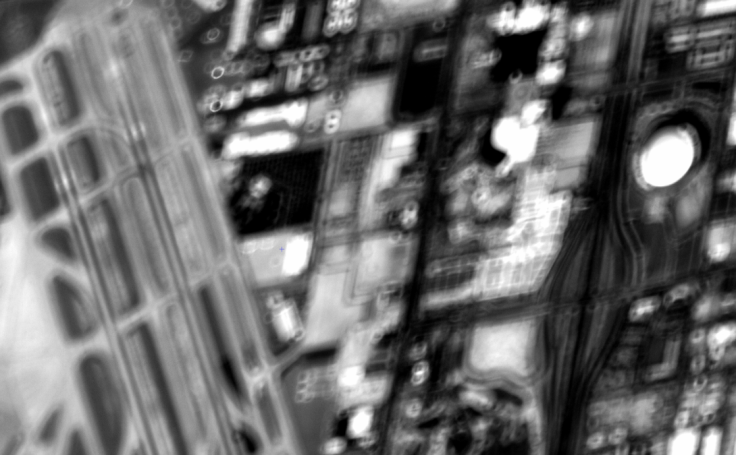
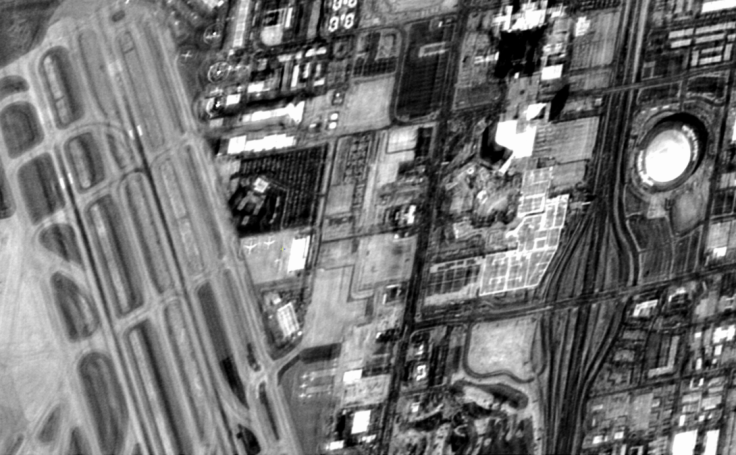
As the space industry accelerates its shift toward small, affordable satellites known as CubeSats, it's running into a major snag: data quality. These pint-sized spacecraft, often deployed by startups, universities, or developing nations, promise to democratize access to Earth Observation (EO). But more often than not, their promise is grounded in technical setbacks, most notably, corrupted or blurry imagery that makes the data unusable.
"Imagine spending hundreds of thousands of dollars to launch a satellite, only to receive imagery where you can't even recognize a plane sitting on a runway," says Wolfgang Lück, Managing Director of EOIntelligence. "That's what's happening. And in most cases, the data gets written off, which means the satellite is written off."
Lück, who has five decades of expertise in the space sector, wants to change that. That is why he has built a solution that's gaining traction across the global EO ecosystem: the Image Resource Management Intelligence (IRMI) system. More than just a tool for fixing out-of-focus satellite images, IRMI is a sophisticated data processing platform designed to transform flawed, fragmented, and inconsistent satellite imagery into what's known as 'analysis-ready data' (ARD). This makes it usable not just by humans but also by machines and AI systems that are capable of extracting insights at scale. At a time when AI is poised to transform everything from agriculture to public health, making satellite data clean, standardized, and machine-readable has become imperative.
CubeSats are revolutionizing Earth observation. They're smaller, more economical, and faster to deploy than traditional satellites. However, their cost-effectiveness often comes at the expense of image quality. Problems with packaging, calibration, and geometric or radiometric accuracy are common.
Under the Committee on Earth Observation Satellites (CEOS) standards, high-quality data must meet ARD criteria and be corrected for atmosphere, topographic, and sensor-specific errors. Most CubeSats fall short of this benchmark, meaning their data is inconsistent or even unusable. "Right now, if a CubeSat returns low-quality imagery, the mission is often deemed a failure," says Lück.
But, where others see scrap, IRMI sees signals. The software identifies errors, corrects atmospheric and topographic distortions, and aligns the data for compatibility with AI systems automatically and at scale. IRMI automates the complex process of transforming satellite raw data into clean, calibrated, CEOS-ARD-compliant imagery. It's built to handle the quirks of small satellite sensors and bring their data up to professional standards.
Lück's obsession with data usability dates back to 1998 when he was a forestry student helping build a university satellite. "I was interested in optimizing spatial problems: how to design roads through a forest based on soil moisture, fire risk, and even bird nesting patterns," he recalls. "The idea was to combine satellite data with ground sensors, weather models, and even market prices to make smart, ecological decisions."
That dream has evolved into IRMI: a sophisticated sensor web that ingests Earth observation measurements from satellites, ground-based monitors, and even unconventional sources like social media or stock exchanges. The system processes this data into a multidimensional 'data cube' with built-in uncertainty metrics, offering analysts the confidence to act on AI-generated insights.
From this architecture springs a cascade of real-world applications. Imagine, in agriculture, IRMI can detect early crop stress, recommend drought-resistant varieties, and advise on irrigation schedules. In disaster management, this software can cue satellites and sensors to zero in on areas showing signs of seismic activity, flooding, or wildfires, often before first responders are even dispatched. In public health, this software can correlate air and water quality with hospital data to predict disease outbreaks.
"It's all about fusion," Lück explains. "Combining high-res optical imagery, coarse but frequent climate data, hyperspectral signals, and more, each with different resolutions and refresh rates, and making that mess usable. Once the data is normalized and fed into our cube, AI takes it from there."
While many tools in the Earth observation sector offer piecemeal solutions, IRMI's strength lies in its unification. It can align 400-band hyperspectral data with three-band RGB imagery or blend satellite-based methane detection with IoT sensor data monitoring pipeline pressure. With CEOS-ARD compliance at its core, the system ensures all this data is not just accurate but analysis-ready.
For small satellite companies struggling with data quality, IRMI offers a lifeline. For governments and NGOs tackling climate change, it's a new frontier of actionable intelligence. And for Lück, it's a way to prove that innovation in space doesn't have to come at the cost of reliability.
"We're at the beginning of something big," he says. "CubeSats have tremendous potential to address the Sustainable Development Goals—from hunger to disaster resilience to climate monitoring. But only if we can trust their data." That trust, Lück argues, starts with validation. And with IRMI, he's building the bridge that connects a fragmented, noisy space ecosystem to the grounded clarity of AI-driven solutions on Earth.
Looking forward, EOIntelligence aims to expand IRMI's capabilities by incorporating new sensor types, such as quantum sensors and next-gen hyperspectral imagers, and exploring partnerships with drone fleets for rapid data collection.
Still, challenges remain. Integrating non-traditional data sources into CEOS-ARD frameworks requires constant innovation, and the computational horsepower needed to process real-time data cubes at a global scale is non-trivial. But if Lück's journey has proven anything, it's that solving hard problems starts with seeing potential in what others dismiss, even if it's just a blurry pixel on a screen.
Originally published on Science Times