How Much Can We Trust AI?
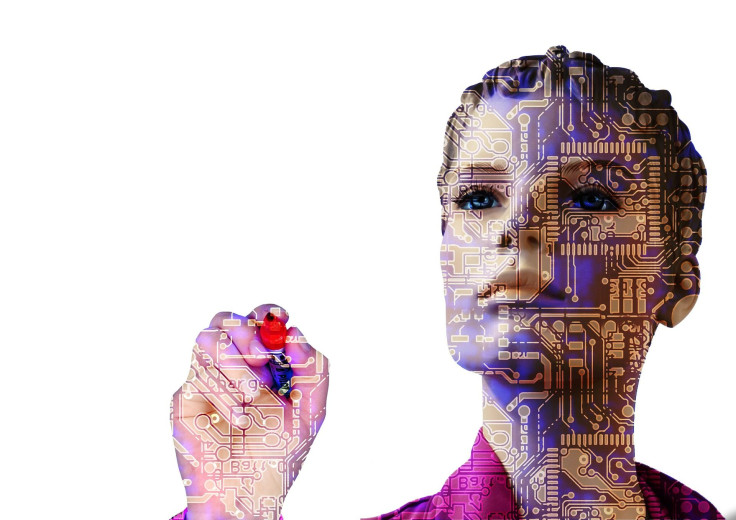
Artificial intelligence software gets more sophisticated almost every day. Computers can already beat human experts at chess, stock market predictions, and detecting cancer. Yet most machine learning experts believe we shouldn’t hand over complete control to AI-powered robots any time soon.
“We don’t trust autonomous vehicles yet, despite the fact that they rarely make mistakes, because the cost of error is too high,” New York University professor Vasant Dhar, who also founded one of the longest running AI-powered hedge funds, told International Business Times. “It takes a while to learn the machine learning program’s style. Just like humans, programs have their own style.”
He told the audience at the Artificial Intelligence & Data Science conference in New York City that the key to trusting AI software is having the right expectations. “I realized this wasn’t a game of finding the ultimate truth of financial markets, but more of finding a process could rely on,” Dhar said. “I started thinking more in terms of a range of expectations for a model. Modeling is a process. It’s never really done. As you get more data, you should be able to learn and improve things.”
In short, machine learning is really good at analyzing huge amounts of data, more information than a human expert could ever manage. AI software is also great at finding patterns and anomalies. “Machine learning is one part of the process: the discovery process,” Dhar said. “There are all kinds of other essential components... There is an incredibly large amount of design choices that go into this process.”
So we can trust robots have lots of processing power and laser vision, although they still need humans to guide the machine’s training. Pavan Arora, head of data at IBM Watson, told IBT the blockchain boom currently sweeping the tech industry will have dramatic impacts on AI capabilities as well, especially when it comes to finance.
“We’re talking about revolutionary transparency,” Arora told IBT. “You can set up your own private blockchain with Watson...that’s what we’re working towards. A cohesive solution for our clients that creates, not only operational efficiency, but also much more accurate data and transparency around that data for everything from inventory management to driving new insights.”
Forbes reported IBM Watson’s AI tools will support 20,000 employees across 5,000 branches of the French bank Crédit Mutuel. And that’s just one client. Arora believes combining machine learning with blockchain technology could also help address one of the fintech industry’s biggest pain points: compliance.
Compliance is one of the biggest challenges facing both the people making new financial products and traditional organizations eager to work with blockchain-based assets such as cryptocurrencies. Watson’s AI tools could help analyze more legal documents than human lawyers ever could on their own. As such, these deep learning tools can boost clarity and alleviate a lot of the legal team’s grunt work. “What if Watson could give an understanding about how my product correlates to obligations, which Watson can extract from the regulations,” Arora said. “It’s called RCA, regulation compliance analysis... regulations in finance are, I think, one of the greatest opportunities for Watson to provide efficiency.”
Michael Beal, JPMorgan alum and CEO of the AI-centric hedge fund Data Capital Management, told IBT 2018 could be the “tipping point” for machine learning. “These technologies are really advancing. They are no longer theoretical. There are a lot of use cases out there where this is adding extreme value,” Beal said. “Now we are getting to a point where there is five to 10 years of history [for alternative data sets]...what you didn’t have before was a long history of unstructured variables. The satellite imagery, the news analysis, the unstructured data.”
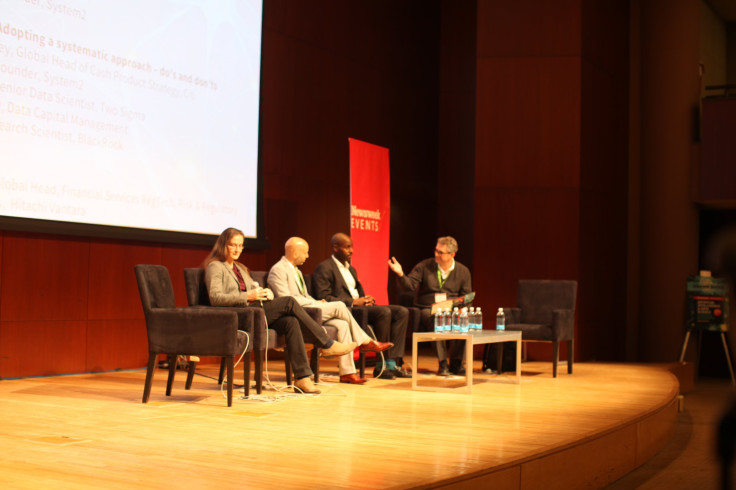
Alternative data analysis is an increasingly lucrative business. International Data Corp. reported AI solutions are now a $12.5 billion industry, expected to garner $46 billion in annual revenues by 2020. Whether machine learning tools are processing geolocation data or crowdsourced algorithms and open source software, many are now working with some of the most diverse data sets in the history of computing. Beal expects AI technologies will reach a point, just like an athlete in training, where the uphill battle to learn breaks through into the realm of technique and artistry. “There’s a tremendous amount, not just of new data, but also the history of that new data,” Beal said. “The longer history of that new data is much more fertile grounds for machine learning.”
Robots are smartest when they have longer records to analyze. Will we someday trust machines to make smarter choices than humans, not simply carry out functions and find better options? Beal believes so. “For me, it’s not a matter of if,” he said. “There will come a point when there is enough information that the machine learning says: that’s enough. Nobody else is beating me...in the financial services market, maybe five plus years worth of data will be enough for that tipping point to occur."
Editor's note: Newsweek Media Group and International Business Times partnered with Structure to host this week's Artificial Intelligence & Data Science event.
© Copyright IBTimes 2024. All rights reserved.